Unsupervised Monocular Depth Learning with Integrated Intrinsics and Spatio-Temporal Constraints
K. Chen, A. Pogue, B.T. Lopez, A. Agha-mohammadi, and A. Mehta, “Unsupervised Monocular Depth Learning with Integrated Intrinsics and Spatio-Temporal Constraints,” IEEE/RSJ International Conference on Intelligent Robots and Systems (IROS), Prague, Czech Republic, Oct 2021, pp. 2451-2458, doi: 10.1109/IROS51168.2021.9636030.
Paper: https://ieeexplore.ieee.org/document/9636030
Video: https://www.youtube.com/watch?v=pu5Z58a5_Xw
Abstract
Monocular depth inference has gained tremendous attention from researchers in recent years and remains as a promising replacement for expensive time-of-flight sensors, but issues with scale acquisition and implementation overhead still plague these systems. To this end, this work presents an unsupervised learning framework that is able to predict at-scale depth maps and egomotion, in addition to camera intrinsics, from a sequence of monocular images via a single network. Our method incorporates both spatial and temporal geometric constraints to resolve depth and pose scale factors, which are enforced within the supervisory reconstruction loss functions at training time. Only unlabeled stereo sequences are required for training the weights of our single-network architecture, which reduces overall implementation overhead as compared to previous methods. Our results demonstrate strong performance when compared to the current state-of-the-art on multiple sequences of the KITTI driving dataset.
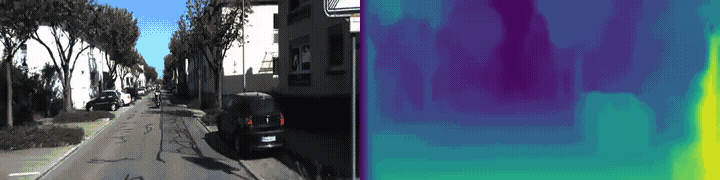
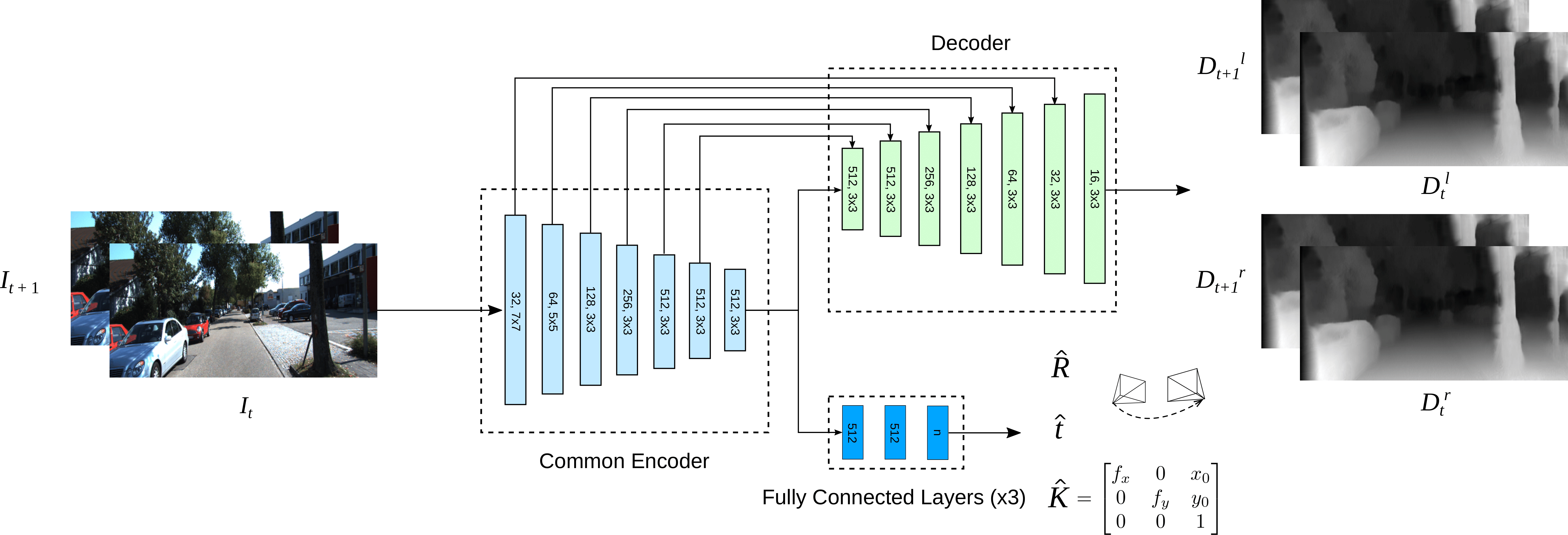
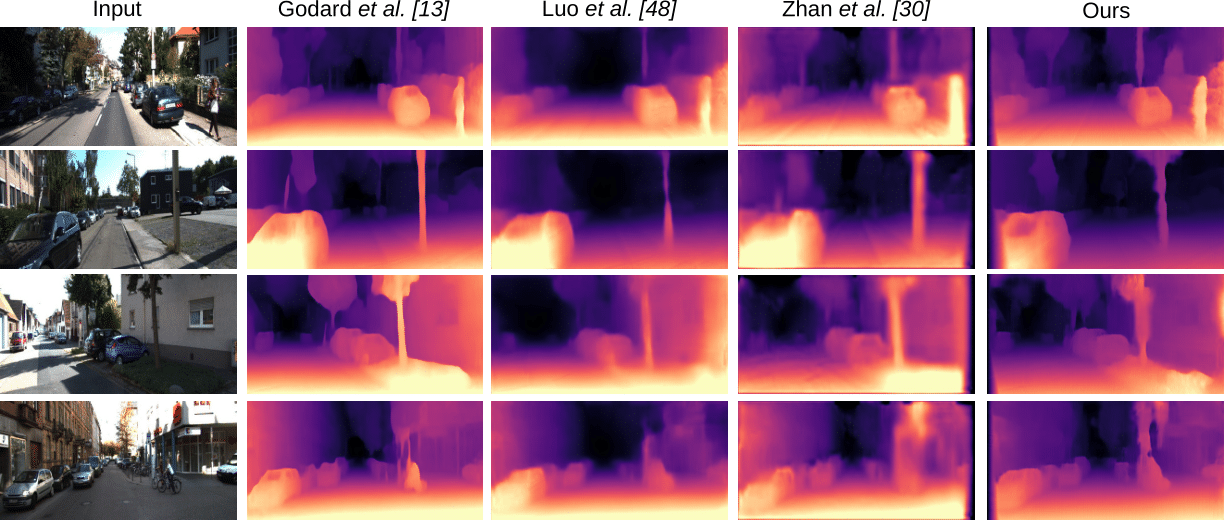